This article has been updated with advanced strategies for improving demand forecasting accuracy. Links to five related articles provide additional insights on inventory optimization, reverse logistics, and balancing overstock and stockouts, ensuring businesses can respond to fluctuating customer demand effectively.

Demand forecasting is the cornerstone of effective inventory management. Businesses that fail to accurately predict customer demand risk stockouts, overstock, or both—all of which can result in financial losses and dissatisfied customers. By adopting the right tools and strategies, companies can improve their demand planning, streamline operations, and ensure they have the inventory needed to meet customer expectations.
Explore foundational inventory strategies in Essential Elements of an Effective Inventory Management Strategy.
Strategies for Improving Demand Forecasting
- Utilize Advanced Forecasting Tools
Demand forecasting tools powered by machine learning and artificial intelligence analyze past sales data, market trends, and external factors to predict future demand. These tools enable businesses to adjust their inventory flow dynamically, ensuring they have the right products at the right time.
Discover how accurate forecasting can minimize stockouts and maximize profits in Inventory Management: How to Avoid Overstock and Stockouts While Maximizing Profits.
- Integrate Real-Time Inventory Visibility
Real-time inventory visibility systems provide businesses with the data they need to make proactive decisions. These systems allow companies to track stock levels, monitor demand fluctuations, and respond quickly to changes in customer preferences.
Learn how to boost inventory visibility in Key Inventory Management Terms.
- Account for Seasonal Trends and External Factors
Seasonal demand patterns, holidays, and external disruptions like supply chain constraints or economic shifts can all impact demand. Incorporating these variables into forecasting models ensures that inventory levels align with anticipated spikes or slow periods.
Read about strategies for avoiding excess inventory and stockouts in Increasing Profitability Through Inventory Optimization.
- Align Demand Forecasting with Reverse Logistics
Returned items are often overlooked in demand forecasts but can significantly impact inventory planning. Efficient reverse logistics processes ensure returned goods are assessed, restocked, or disposed of in a way that aligns with current demand forecasts.
Explore how reverse logistics integrates with demand forecasting in How to Optimize Your Reverse Logistics Operations.
- Leverage Returns Management Systems for Insights
Returns management systems (RMS) provide critical data on customer behavior, such as why products are returned. This information can be used to refine demand forecasts, ensuring businesses can better predict product returns and plan inventory accordingly.
Learn more about RMS in Returns Management Systems and Digital Supply Networks are Critical for Success in Today’s Disruptive World.
The Role of Demand Forecasting in Inventory Management
Improved demand forecasting enables businesses to respond to changing customer needs with precision. By leveraging advanced tools, real-time visibility, and insights from reverse logistics and RMS, companies can achieve optimal inventory levels and minimize costs.
For further insights into demand forecasting and inventory management, explore the resources linked throughout this article.
How can we help improve your supply chain operations?
Schedule a consultation or contact Tompkins Solutions for more information.
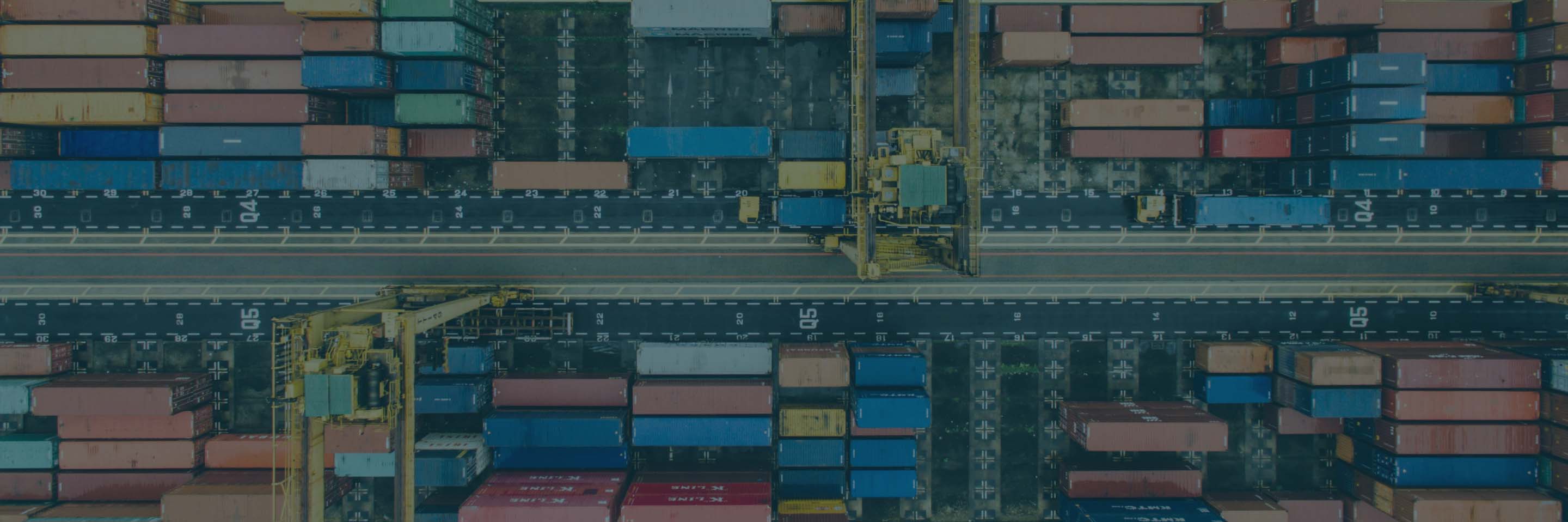
Featured Posts
Discover valuable resources to enhance your knowledge.